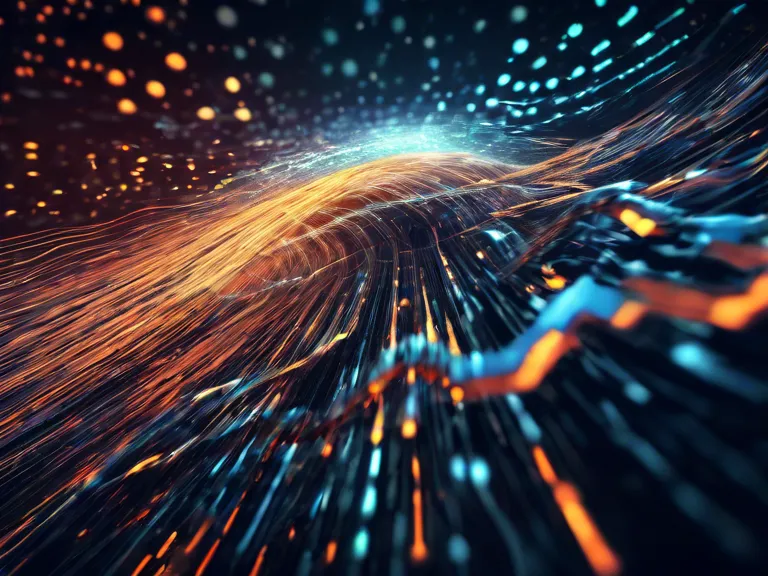
In the fast-paced world of data science, speed is of the essence. Rapid data processing is the future of data science, enabling organizations to quickly analyze and derive insights from vast amounts of data. With advancements in technology and machine learning algorithms, the speed at which data can be processed is increasing at an unprecedented rate. In this article, we will explore the importance of rapid data processing in data science and its implications for the future.
One of the key benefits of rapid data processing is the ability to make real-time decisions. In industries such as finance, healthcare, and e-commerce, time is of the essence when it comes to making critical decisions. With rapid data processing, organizations can quickly analyze data streams and respond to changing conditions in real-time. This can give them a competitive edge and help them stay ahead of the curve.
Another benefit of rapid data processing is the ability to analyze large volumes of data quickly. With the exponential growth of data in recent years, traditional data processing methods are no longer sufficient. Rapid data processing techniques such as in-memory computing and parallel processing allow organizations to analyze massive datasets in a fraction of the time it would take using traditional methods. This enables them to uncover hidden patterns and insights that can drive business growth.
Furthermore, rapid data processing can also improve the accuracy of data analysis. By processing data quickly, organizations can reduce the risk of errors and make more informed decisions. Machine learning algorithms can be trained on large datasets in a short amount of time, leading to more accurate predictions and insights.
In conclusion, rapid data processing is the future of speed in data science. Organizations that can harness the power of rapid data processing will be able to make real-time decisions, analyze large volumes of data quickly, and improve the accuracy of their data analysis. By embracing rapid data processing techniques, organizations can stay ahead of the competition and drive innovation in the ever-evolving field of data science.